Chapter 10 Chimeric mouse embryo (10X Genomics)
10.1 Introduction
This performs an analysis of the Pijuan-Sala et al. (2019) dataset on mouse gastrulation. Here, we examine chimeric embryos at the E8.5 stage of development where td-Tomato-positive embryonic stem cells (ESCs) were injected into a wild-type blastocyst.
10.2 Data loading
## class: SingleCellExperiment
## dim: 29453 20935
## metadata(0):
## assays(1): counts
## rownames(29453): ENSMUSG00000051951 ENSMUSG00000089699 ...
## ENSMUSG00000095742 tomato-td
## rowData names(2): ENSEMBL SYMBOL
## colnames(20935): cell_9769 cell_9770 ... cell_30702 cell_30703
## colData names(11): cell barcode ... doub.density sizeFactor
## reducedDimNames(2): pca.corrected.E7.5 pca.corrected.E8.5
## mainExpName: NULL
## altExpNames(0):
10.3 Quality control
Quality control on the cells has already been performed by the authors, so we will not repeat it here. We additionally remove cells that are labelled as stripped nuclei or doublets.
10.5 Variance modelling
We retain all genes with any positive biological component, to preserve as much signal as possible across a very heterogeneous dataset.
library(scran)
dec.chimera <- modelGeneVar(sce.chimera, block=sce.chimera$sample)
chosen.hvgs <- dec.chimera$bio > 0
par(mfrow=c(1,2))
blocked.stats <- dec.chimera$per.block
for (i in colnames(blocked.stats)) {
current <- blocked.stats[[i]]
plot(current$mean, current$total, main=i, pch=16, cex=0.5,
xlab="Mean of log-expression", ylab="Variance of log-expression")
curfit <- metadata(current)
curve(curfit$trend(x), col='dodgerblue', add=TRUE, lwd=2)
}
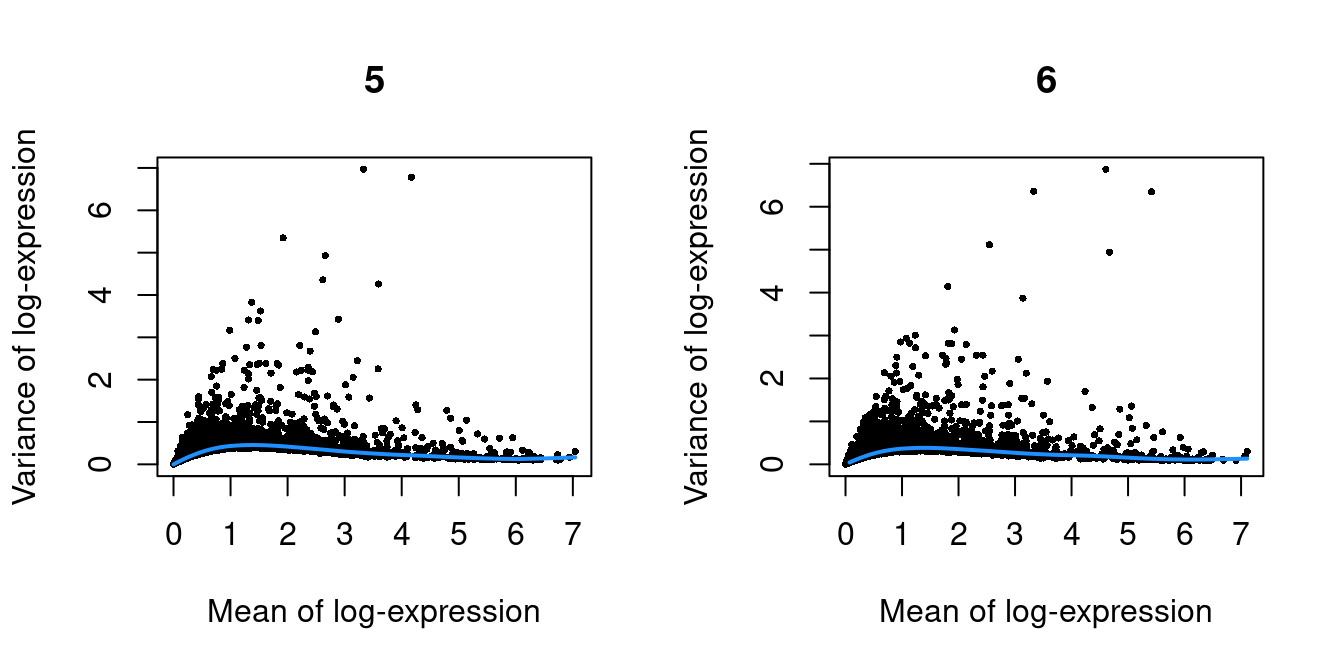
Figure 10.1: Per-gene variance as a function of the mean for the log-expression values in the Pijuan-Sala chimeric mouse embryo dataset. Each point represents a gene (black) with the mean-variance trend (blue) fitted to the variances.
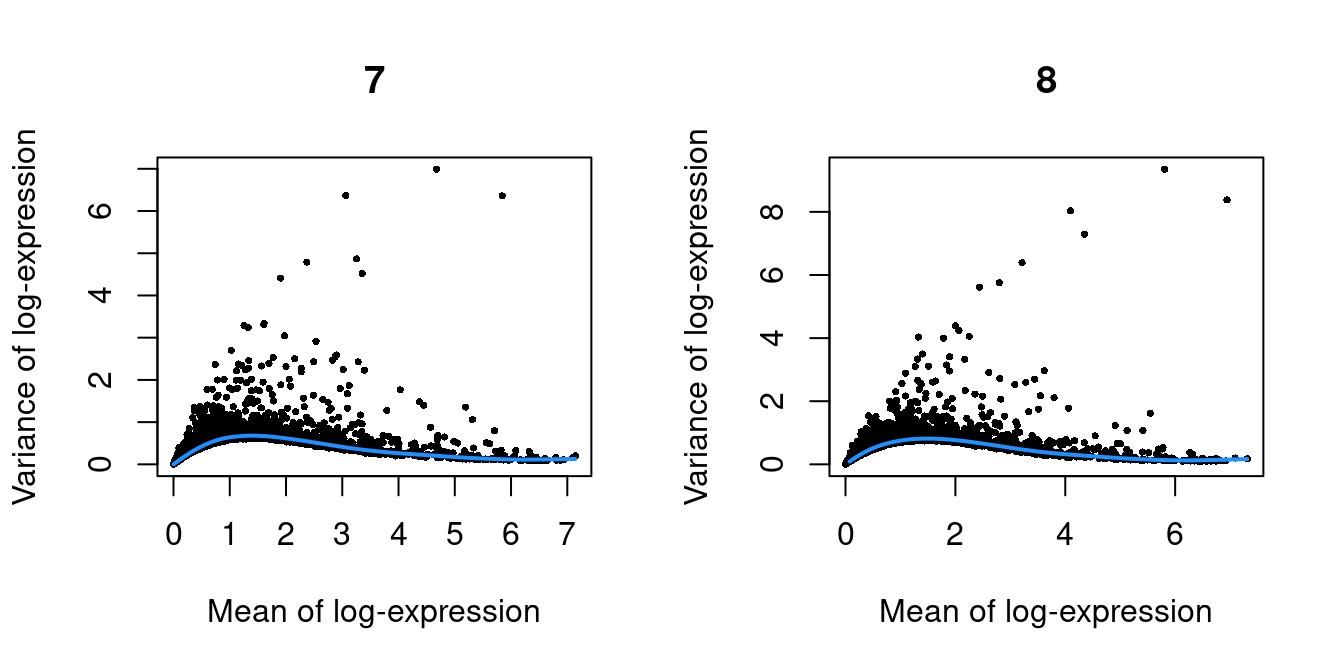
Figure 10.2: Per-gene variance as a function of the mean for the log-expression values in the Pijuan-Sala chimeric mouse embryo dataset. Each point represents a gene (black) with the mean-variance trend (blue) fitted to the variances.
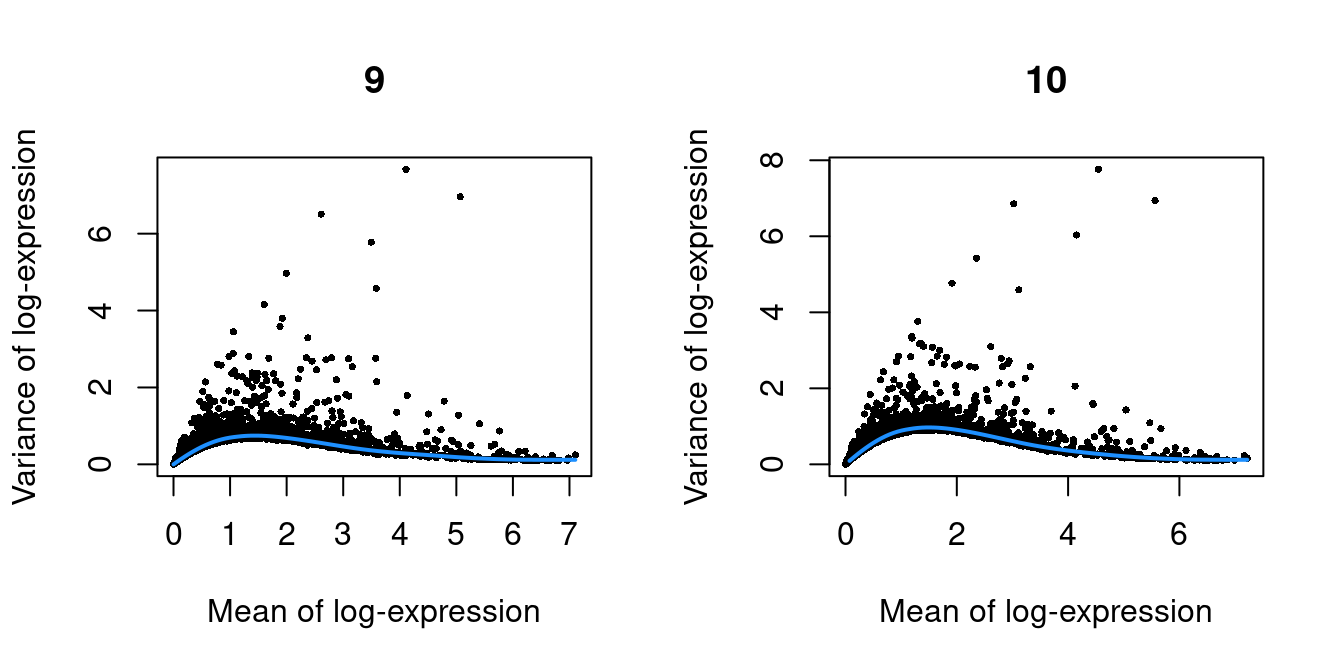
Figure 10.3: Per-gene variance as a function of the mean for the log-expression values in the Pijuan-Sala chimeric mouse embryo dataset. Each point represents a gene (black) with the mean-variance trend (blue) fitted to the variances.
10.6 Merging
We use a hierarchical merge to first merge together replicates with the same genotype, and then merge samples across different genotypes.
library(batchelor)
set.seed(01001001)
merged <- correctExperiments(sce.chimera,
batch=sce.chimera$sample,
subset.row=chosen.hvgs,
PARAM=FastMnnParam(
merge.order=list(
list(1,3,5), # WT (3 replicates)
list(2,4,6) # td-Tomato (3 replicates)
)
)
)
We use the percentage of variance lost as a diagnostic:
## 5 6 7 8 9 10
## [1,] 0.000e+00 0.0204433 0.000e+00 0.0169567 0.000000 0.000000
## [2,] 0.000e+00 0.0007389 0.000e+00 0.0004409 0.000000 0.015474
## [3,] 3.090e-02 0.0000000 2.012e-02 0.0000000 0.000000 0.000000
## [4,] 9.024e-05 0.0000000 8.272e-05 0.0000000 0.018047 0.000000
## [5,] 4.321e-03 0.0072518 4.124e-03 0.0078280 0.003831 0.007786
10.7 Clustering
g <- buildSNNGraph(merged, use.dimred="corrected")
clusters <- igraph::cluster_louvain(g)
colLabels(merged) <- factor(clusters$membership)
We examine the distribution of cells across clusters and samples.
## Sample
## Cluster 5 6 7 8 9 10
## 1 77 19 56 50 131 60
## 2 148 37 133 110 231 216
## 3 98 16 165 128 369 273
## 4 185 115 328 593 460 547
## 5 135 72 322 594 296 778
## 6 212 53 344 203 536 612
## 7 149 73 86 86 163 383
## 8 133 97 110 66 162 313
## 9 84 21 79 35 170 213
## 10 174 45 219 182 211 381
## 11 97 19 36 18 50 35
## 12 111 41 45 35 40 147
## 13 123 64 62 51 63 140
## 14 157 78 130 104 164 436
## 15 110 69 72 96 127 253
## 16 43 35 82 80 85 354
## 17 77 43 191 118 329 487
## 18 47 22 82 51 87 130
## 19 39 41 50 48 128 125
## 20 1 5 0 84 0 66
## 21 18 7 13 17 20 37
## 22 58 29 90 79 81 188
## 23 9 7 18 13 30 27
## 24 11 15 20 9 47 57
## 25 2 1 7 3 77 138
## 26 0 2 0 51 0 5
10.8 Dimensionality reduction
We use an external algorithm to compute nearest neighbors for greater speed.
merged <- runTSNE(merged, dimred="corrected", external_neighbors=TRUE)
merged <- runUMAP(merged, dimred="corrected", external_neighbors=TRUE)
gridExtra::grid.arrange(
plotTSNE(merged, colour_by="label", text_by="label", text_colour="red"),
plotTSNE(merged, colour_by="batch")
)
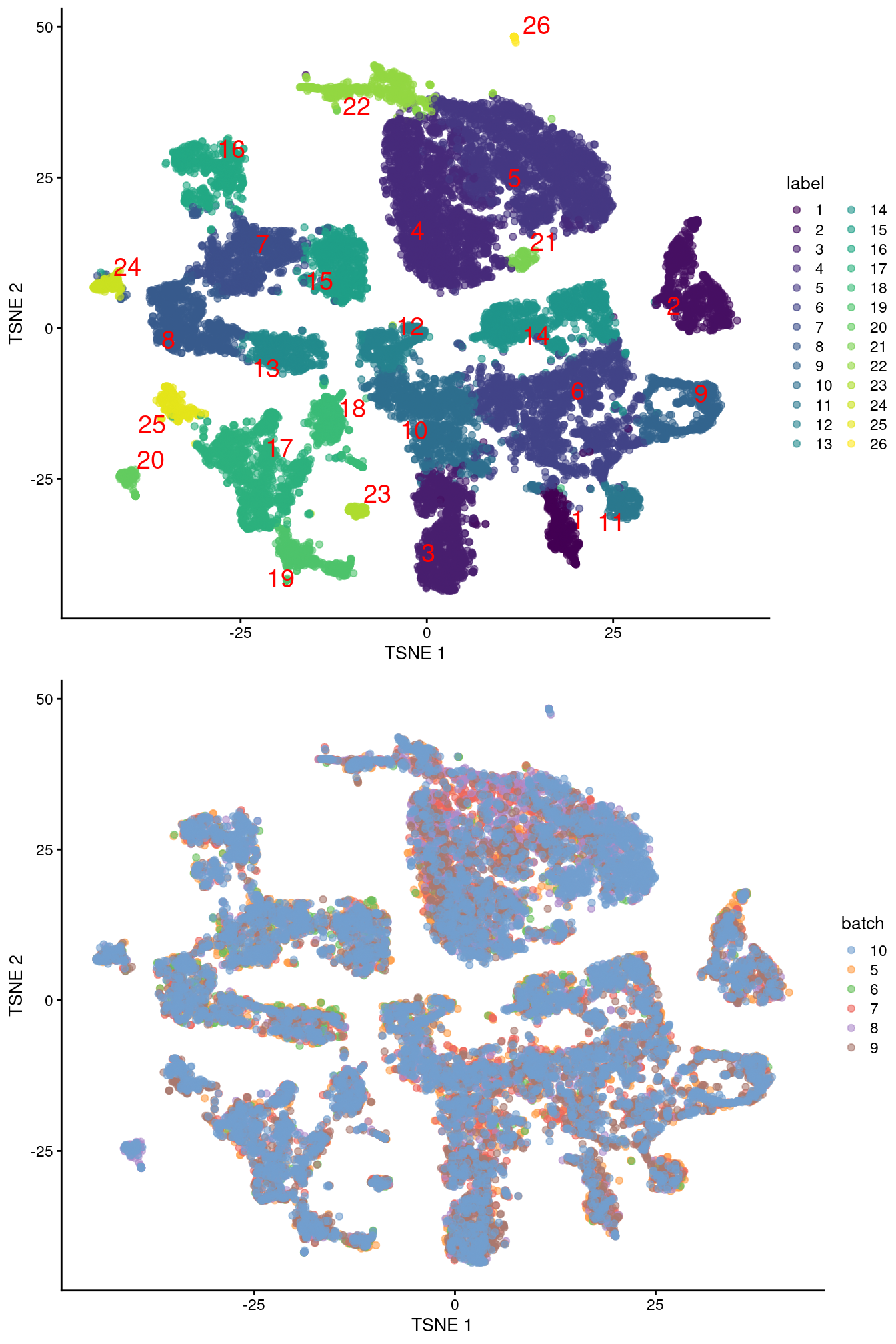
Figure 10.4: Obligatory \(t\)-SNE plots of the Pijuan-Sala chimeric mouse embryo dataset, where each point represents a cell and is colored according to the assigned cluster (top) or sample of origin (bottom).
Session Info
R version 4.3.0 RC (2023-04-13 r84269)
Platform: x86_64-pc-linux-gnu (64-bit)
Running under: Ubuntu 22.04.2 LTS
Matrix products: default
BLAS: /home/biocbuild/bbs-3.17-bioc/R/lib/libRblas.so
LAPACK: /usr/lib/x86_64-linux-gnu/lapack/liblapack.so.3.10.0
locale:
[1] LC_CTYPE=en_US.UTF-8 LC_NUMERIC=C
[3] LC_TIME=en_GB LC_COLLATE=C
[5] LC_MONETARY=en_US.UTF-8 LC_MESSAGES=en_US.UTF-8
[7] LC_PAPER=en_US.UTF-8 LC_NAME=C
[9] LC_ADDRESS=C LC_TELEPHONE=C
[11] LC_MEASUREMENT=en_US.UTF-8 LC_IDENTIFICATION=C
time zone: America/New_York
tzcode source: system (glibc)
attached base packages:
[1] stats4 stats graphics grDevices utils datasets methods
[8] base
other attached packages:
[1] batchelor_1.16.0 scran_1.28.1
[3] scater_1.28.0 ggplot2_3.4.2
[5] scuttle_1.10.1 MouseGastrulationData_1.14.0
[7] SpatialExperiment_1.10.0 SingleCellExperiment_1.22.0
[9] SummarizedExperiment_1.30.1 Biobase_2.60.0
[11] GenomicRanges_1.52.0 GenomeInfoDb_1.36.0
[13] IRanges_2.34.0 S4Vectors_0.38.1
[15] BiocGenerics_0.46.0 MatrixGenerics_1.12.0
[17] matrixStats_0.63.0 BiocStyle_2.28.0
[19] rebook_1.10.0
loaded via a namespace (and not attached):
[1] jsonlite_1.8.4 CodeDepends_0.6.5
[3] magrittr_2.0.3 ggbeeswarm_0.7.2
[5] magick_2.7.4 farver_2.1.1
[7] rmarkdown_2.21 zlibbioc_1.46.0
[9] vctrs_0.6.2 memoise_2.0.1
[11] DelayedMatrixStats_1.22.0 RCurl_1.98-1.12
[13] htmltools_0.5.5 S4Arrays_1.0.4
[15] AnnotationHub_3.8.0 curl_5.0.0
[17] BiocNeighbors_1.18.0 Rhdf5lib_1.22.0
[19] rhdf5_2.44.0 sass_0.4.6
[21] bslib_0.4.2 cachem_1.0.8
[23] ResidualMatrix_1.10.0 igraph_1.4.2
[25] mime_0.12 lifecycle_1.0.3
[27] pkgconfig_2.0.3 rsvd_1.0.5
[29] Matrix_1.5-4 R6_2.5.1
[31] fastmap_1.1.1 GenomeInfoDbData_1.2.10
[33] shiny_1.7.4 digest_0.6.31
[35] colorspace_2.1-0 AnnotationDbi_1.62.1
[37] dqrng_0.3.0 irlba_2.3.5.1
[39] ExperimentHub_2.8.0 RSQLite_2.3.1
[41] beachmat_2.16.0 labeling_0.4.2
[43] filelock_1.0.2 fansi_1.0.4
[45] httr_1.4.6 compiler_4.3.0
[47] bit64_4.0.5 withr_2.5.0
[49] BiocParallel_1.34.1 viridis_0.6.3
[51] DBI_1.1.3 highr_0.10
[53] HDF5Array_1.28.1 R.utils_2.12.2
[55] rappdirs_0.3.3 DelayedArray_0.26.2
[57] bluster_1.10.0 rjson_0.2.21
[59] tools_4.3.0 vipor_0.4.5
[61] beeswarm_0.4.0 interactiveDisplayBase_1.38.0
[63] httpuv_1.6.11 R.oo_1.25.0
[65] glue_1.6.2 rhdf5filters_1.12.1
[67] promises_1.2.0.1 grid_4.3.0
[69] Rtsne_0.16 cluster_2.1.4
[71] generics_0.1.3 gtable_0.3.3
[73] R.methodsS3_1.8.2 metapod_1.8.0
[75] BiocSingular_1.16.0 ScaledMatrix_1.8.1
[77] utf8_1.2.3 XVector_0.40.0
[79] ggrepel_0.9.3 BiocVersion_3.17.1
[81] pillar_1.9.0 limma_3.56.1
[83] BumpyMatrix_1.8.0 later_1.3.1
[85] dplyr_1.1.2 BiocFileCache_2.8.0
[87] lattice_0.21-8 bit_4.0.5
[89] tidyselect_1.2.0 locfit_1.5-9.7
[91] Biostrings_2.68.1 knitr_1.42
[93] gridExtra_2.3 bookdown_0.34
[95] edgeR_3.42.2 xfun_0.39
[97] statmod_1.5.0 DropletUtils_1.20.0
[99] yaml_2.3.7 evaluate_0.21
[101] codetools_0.2-19 tibble_3.2.1
[103] BiocManager_1.30.20 graph_1.78.0
[105] cli_3.6.1 uwot_0.1.14
[107] xtable_1.8-4 munsell_0.5.0
[109] jquerylib_0.1.4 Rcpp_1.0.10
[111] dir.expiry_1.8.0 dbplyr_2.3.2
[113] png_0.1-8 XML_3.99-0.14
[115] parallel_4.3.0 ellipsis_0.3.2
[117] blob_1.2.4 sparseMatrixStats_1.12.0
[119] bitops_1.0-7 viridisLite_0.4.2
[121] scales_1.2.1 purrr_1.0.1
[123] crayon_1.5.2 rlang_1.1.1
[125] cowplot_1.1.1 KEGGREST_1.40.0
Butler, A., P. Hoffman, P. Smibert, E. Papalexi, and R. Satija. 2018. “Integrating single-cell transcriptomic data across different conditions, technologies, and species.” Nat. Biotechnol. 36 (5): 411–20.
Büttner, Maren, Zhichao Miao, F Alexander Wolf, Sarah A Teichmann, and Fabian J Theis. 2019. “A Test Metric for Assessing Single-Cell Rna-Seq Batch Correction.” Nature Methods 16 (1): 43–49.
Chen, Y., A. T. Lun, and G. K. Smyth. 2016. “From reads to genes to pathways: differential expression analysis of RNA-Seq experiments using Rsubread and the edgeR quasi-likelihood pipeline.” F1000Res 5: 1438.
Crowell, H. L., C. Soneson, P.-L. Germain, D. Calini, L. Collin, C. Raposo, D. Malhotra, and M. D. Robinson. 2019. “On the Discovery of Population-Specific State Transitions from Multi-Sample Multi-Condition Single-Cell Rna Sequencing Data.” bioRxiv. https://doi.org/10.1101/713412.
Finak, G., J. Frelinger, W. Jiang, E. W. Newell, J. Ramey, M. M. Davis, S. A. Kalams, S. C. De Rosa, and R. Gottardo. 2014. “OpenCyto: an open source infrastructure for scalable, robust, reproducible, and automated, end-to-end flow cytometry data analysis.” PLoS Comput. Biol. 10 (8): e1003806.
Grun, D., M. J. Muraro, J. C. Boisset, K. Wiebrands, A. Lyubimova, G. Dharmadhikari, M. van den Born, et al. 2016. “De Novo Prediction of Stem Cell Identity using Single-Cell Transcriptome Data.” Cell Stem Cell 19 (2): 266–77.
Haghverdi, L., A. T. L. Lun, M. D. Morgan, and J. C. Marioni. 2018. “Batch effects in single-cell RNA-sequencing data are corrected by matching mutual nearest neighbors.” Nat. Biotechnol. 36 (5): 421–27.
Lawlor, N., J. George, M. Bolisetty, R. Kursawe, L. Sun, V. Sivakamasundari, I. Kycia, P. Robson, and M. L. Stitzel. 2017. “Single-cell transcriptomes identify human islet cell signatures and reveal cell-type-specific expression changes in type 2 diabetes.” Genome Res. 27 (2): 208–22.
Leek, J. T., W. E. Johnson, H. S. Parker, A. E. Jaffe, and J. D. Storey. 2012. “The sva package for removing batch effects and other unwanted variation in high-throughput experiments.” Bioinformatics 28 (6): 882–83.
Lin, Y., S. Ghazanfar, K. Y. X. Wang, J. A. Gagnon-Bartsch, K. K. Lo, X. Su, Z. G. Han, et al. 2019. “scMerge leverages factor analysis, stable expression, and pseudoreplication to merge multiple single-cell RNA-seq datasets.” Proc. Natl. Acad. Sci. U.S.A. 116 (20): 9775–84.
Lun, A., S. Riesenfeld, T. Andrews, T. P. Dao, T. Gomes, participants in the 1st Human Cell Atlas Jamboree, and J. Marioni. 2019. “EmptyDrops: distinguishing cells from empty droplets in droplet-based single-cell RNA sequencing data.” Genome Biol. 20 (1): 63.
Lun, A. T. L., and J. C. Marioni. 2017. “Overcoming confounding plate effects in differential expression analyses of single-cell RNA-seq data.” Biostatistics 18 (3): 451–64.
Lun, A. T. L., A. C. Richard, and J. C. Marioni. 2017. “Testing for differential abundance in mass cytometry data.” Nat. Methods 14 (7): 707–9.
McCarthy, D. J., and G. K. Smyth. 2009. “Testing significance relative to a fold-change threshold is a TREAT.” Bioinformatics 25 (6): 765–71.
Muraro, M. J., G. Dharmadhikari, D. Grun, N. Groen, T. Dielen, E. Jansen, L. van Gurp, et al. 2016. “A Single-Cell Transcriptome Atlas of the Human Pancreas.” Cell Syst 3 (4): 385–94.
Phipson, B., S. Lee, I. J. Majewski, W. S. Alexander, and G. K. Smyth. 2016. “Robust Hyperparameter Estimation Protects Against Hypervariable Genes and Improves Power to Detect Differential Expression.” Ann. Appl. Stat. 10 (2): 946–63.
Pijuan-Sala, B., J. A. Griffiths, C. Guibentif, T. W. Hiscock, W. Jawaid, F. J. Calero-Nieto, C. Mulas, et al. 2019. “A Single-Cell Molecular Map of Mouse Gastrulation and Early Organogenesis.” Nature 566 (7745): 490–95.
Richard, A. C., A. T. L. Lun, W. W. Y. Lau, B. Gottgens, J. C. Marioni, and G. M. Griffiths. 2018. “T cell cytolytic capacity is independent of initial stimulation strength.” Nat. Immunol. 19 (8): 849–58.
Ritchie, M. E., B. Phipson, D. Wu, Y. Hu, C. W. Law, W. Shi, and G. K. Smyth. 2015. “limma powers differential expression analyses for RNA-sequencing and microarray studies.” Nucleic Acids Res. 43 (7): e47.
Robinson, M. D., D. J. McCarthy, and G. K. Smyth. 2010. “edgeR: a Bioconductor package for differential expression analysis of digital gene expression data.” Bioinformatics 26 (1): 139–40.
Robinson, M. D., and A. Oshlack. 2010. “A scaling normalization method for differential expression analysis of RNA-seq data.” Genome Biol. 11 (3): R25.
Scialdone, A., Y. Tanaka, W. Jawaid, V. Moignard, N. K. Wilson, I. C. Macaulay, J. C. Marioni, and B. Gottgens. 2016. “Resolving early mesoderm diversification through single-cell expression profiling.” Nature 535 (7611): 289–93.
Segerstolpe, A., A. Palasantza, P. Eliasson, E. M. Andersson, A. C. Andreasson, X. Sun, S. Picelli, et al. 2016. “Single-Cell Transcriptome Profiling of Human Pancreatic Islets in Health and Type 2 Diabetes.” Cell Metab. 24 (4): 593–607.
Tung, P. Y., J. D. Blischak, C. J. Hsiao, D. A. Knowles, J. E. Burnett, J. K. Pritchard, and Y. Gilad. 2017. “Batch effects and the effective design of single-cell gene expression studies.” Sci. Rep. 7 (January): 39921.
Young, M. D., and S. Behjati. 2018. “SoupX Removes Ambient RNA Contamination from Droplet Based Single Cell RNA Sequencing Data.” bioRxiv.
Zheng, G. X., J. M. Terry, P. Belgrader, P. Ryvkin, Z. W. Bent, R. Wilson, S. B. Ziraldo, et al. 2017. “Massively parallel digital transcriptional profiling of single cells.” Nat Commun 8 (January): 14049.
References
Pijuan-Sala, B., J. A. Griffiths, C. Guibentif, T. W. Hiscock, W. Jawaid, F. J. Calero-Nieto, C. Mulas, et al. 2019. “A Single-Cell Molecular Map of Mouse Gastrulation and Early Organogenesis.” Nature 566 (7745): 490–95.